Contents
- 1 Introduction to Master Data in Supply Chain and Manufacturing
- 2 Examples of Master Data
- 3 The Importance of Master Data
- 4 The Consequences of Poor Master Data Management
- 5 Common Challenges in Managing Master Data
- 6 Overcoming the Challenges: Best Practices for Master Data Management
- 7 Conclusion: The Power of Master Data in Transforming Supply Chain and Manufacturing
Introduction to Master Data in Supply Chain and Manufacturing
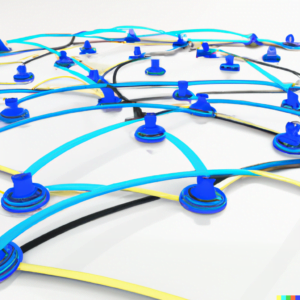
Master Data in Supply Chain and Manufacturing represents the business-critical information that forms the backbone of an organization’s transactional operations and decision-making processes. In the context of supply chain and manufacturing, Master Data encompasses key details about products, suppliers, customers, materials, and facilities. Let’s take the example of a global car manufacturer, the Master Data includes information about:
- Different car models
- Specifications
- Suppliers of various parts
- Manufacturing facilities across the world, and so on.
This information is fundamental to coordinating and executing all supply chain and manufacturing activities, thereby optimizing operational efficiency.
Examples of Master Data
Master Data in supply chain and manufacturing encompasses a variety of data:
Product Information Management | This involves comprehensive details about products, including specifications, production costs, and lifecycle stages. This can be both physical attributes such as dimensions, but also technical attributes that allow the organization to function such as a product hierarchy. For example, a furniture manufacturer’s product data would include information about dimensions, materials used, production time, product classification, and associated costs. |
Supplier and Customer Data | Data about suppliers (such as contact details, lead times, and pricing) and customers (like purchase history and preferences) is critical for managing relationships and predicting demand. So, remember to comply with relevant data privacy requirements! For instance, a beverage company might keep track of suppliers for different ingredients, their prices, and delivery times, to manage production schedules effectively. |
Inventory and Shipment Data | Data about inventory levels, storage locations, shipment schedules, and logistics providers enables efficient inventory management and distribution. Some companies classify this as “planning data”. For instance, an e-commerce company would require real-time data on warehouse inventories and shipping information to ensure prompt order fulfillment. |
The Importance of Master Data
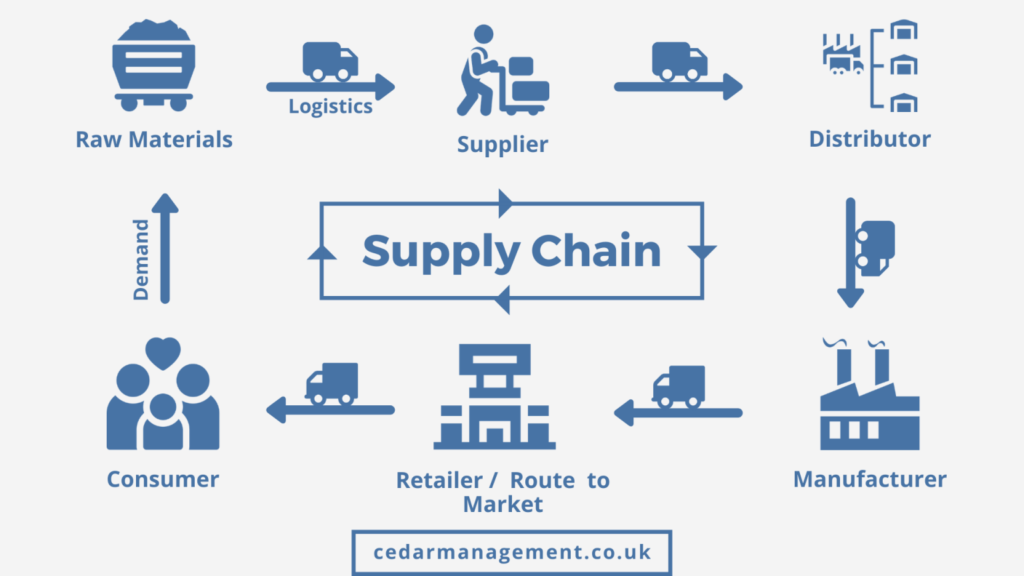
Enhancing Operational Efficiency | Quality Master Data supports efficient operations by eliminating data redundancies, facilitating streamlined data management, and improving the precision of analytics and forecasting. For instance, having accurate and updated data about raw materials, their suppliers, and lead times can help businesses optimize procurement processes, thereby minimizing waste and saving costs. |
Facilitating Better Decision Making | Reliable, accurate Master Data forms the basis for insightful business intelligence that enables strategic decisions. For example, precise data on customer demand and product performance can help leaders in the manufacturing sector determine which product lines to focus on or discontinue. |
Improving Inventory and Production Management | Effective Master Data management ensures accurate tracking of inventory levels and production schedules, preventing overstocking or stockouts and minimizing production delays. For example, with real-time data on inventory levels and sales, a retail company can maintain optimal stock levels, thereby improving customer satisfaction. |
The Consequences of Poor Master Data Management
Ineffective management of Master Data can have severe consequences for businesses, affecting their efficiency, accuracy, and overall profitability. These consequences can manifest in a variety of ways:
Operational Disruptions | Poor Master Data management can lead to operational disruptions. For instance, inaccurate inventory data might lead to stock-outs, disrupting production schedules in a manufacturing firm. Similarly, outdated supplier data could delay the procurement of necessary materials, stalling production lines. |
Reduced Decision-Making Accuracy | With poor quality Master Data, the accuracy of decision-making processes can significantly drop. For example, a logistics firm might miscalculate the required fleet size for transportation if it relies on inaccurate shipment data, leading to over or under-investment in fleet vehicles. |
Financial Losses | Financial losses are often a direct result of operational disruptions and reduced decision-making accuracy. Using the previous example, the logistics firm might face increased costs due to excess fleet maintenance or loss of business due to inadequate fleet size. Similarly, a manufacturing firm dealing with frequent stock-outs might lose out on sales opportunities, impacting its bottom line. |
Damage to Customer Relationships | Inaccurate Master Data can also damage customer relationships. If an e-commerce firm has incorrect product specifications in its database, customers receiving products that do not match their expectations are likely to be dissatisfied, leading to poor reviews and potentially damaging the company’s reputation. |
Non-Compliance with Regulations | In industries where regulatory compliance is crucial, poor Master Data management can lead to non-compliance issues. For instance, in the pharmaceutical industry, inaccurate or incomplete data about drug compositions or clinical trial results can result in regulatory penalties or even product recalls. |
Overall, poor Master Data management can have a significant negative impact on a company’s operations, financial health, and reputation. Therefore, investing in robust Master Data management processes and solutions is vital to avoid these consequences and ensure business success.
Common Challenges in Managing Master Data
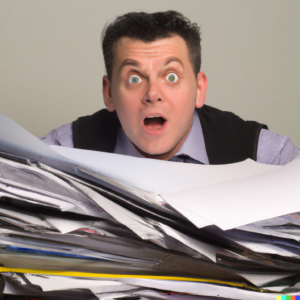
Managing Master Data is often fraught with challenges, impacting its quality and usability. Moreover, these struggles commonly revolve around data quality, data silos, data standardization, change management, and data governance.
Struggles with Data Quality | Erroneous, incomplete, or outdated data can lead to inaccuracies in forecasting and business intelligence, impacting decision-making. For example, incorrect material specifications in a manufacturing company’s Master Data could lead to production errors and product recalls. |
Battling Data Silos | Data silos occur when data is stored separately by different departments, reducing visibility and coordination. For instance, if a pharmaceutical company’s procurement department can’t access real-time inventory data, they might order excess raw materials, leading to increased holding costs. |
The Need for Data Standardization | Lack of standardization can make data integration and analysis challenging. For example, if suppliers provide product data in various formats, a retail company may struggle to consolidate this information, leading to inaccuracies in product listings. |
Change Management in Data Practices | Introducing new data management practices can be met with resistance from employees used to old systems. Without proper change management, the transition can lead to confusion and mistakes, as seen when a manufacturing firm shifts from a legacy system to a modern data management platform without adequate training. |
Establishing Effective Data Governance | Data governance involves defining who is responsible for various data-related tasks and how data should be handled. Poor data governance can lead to confusion, data breaches, and non-compliance with regulations. For instance, without clear data ownership, a logistics company might struggle with managing and securing sensitive shipment data. |
Overcoming the Challenges: Best Practices for Master Data Management
Despite these challenges, companies can adopt several best practices to improve their Master Data management:
Implementing Robust Data Quality Management | Ensure data is accurate, complete, and updated. For example, a manufacturing company could introduce data validation checks to prevent errors in product data entry. |
Breaking Down Data Silos | Promote data sharing and integration across departments to improve visibility and coordination. A retail company could use a centralized data management system accessible to all relevant departments. |
Standardizing Data Entry and Formats | Adopt consistent formats and standards for data entry to facilitate data integration and analysis. For instance, a logistics company could mandate all suppliers to provide shipment data in a specific format. |
Managing Change Effectively | Use change management principles when introducing new data management practices to ensure smooth transitions and employee buy-in. A pharmaceutical company shifting to a new data platform could offer comprehensive training to staff to facilitate the transition. |
Establishing Strong Data Governance | Define clear roles and responsibilities for data-related tasks and establish guidelines for data handling. For example, a global car manufacturer could assign data ownership roles to manage data about different car models. |
Conclusion: The Power of Master Data in Transforming Supply Chain and Manufacturing
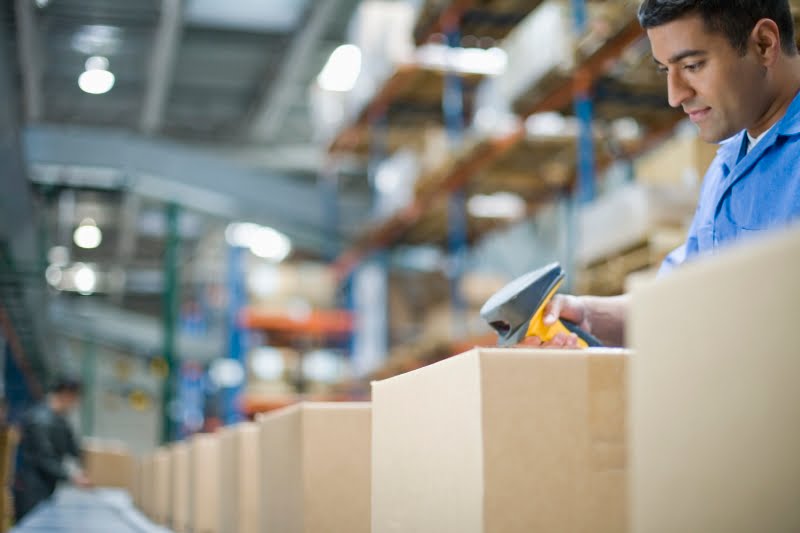
Effective Master Data management is a potent tool that can profoundly transform supply chain and manufacturing operations. Ensuring the quality, consistency, and accessibility of Master Data can lead to enhanced operational efficiency, better decision-making, and greater profitability. So, as we advance in the era of big data and analytics, the significance of Master Data in supply chain and manufacturing will continue to soar.